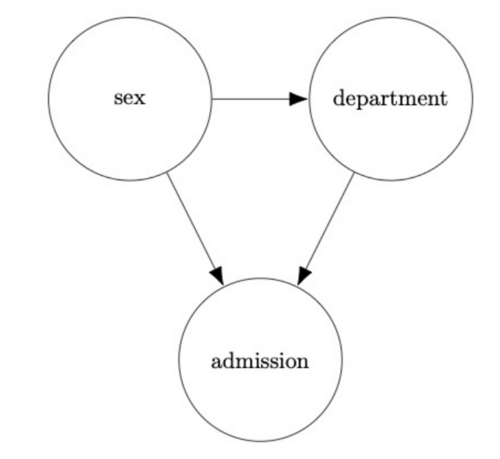
December 28, 2020
Statistical and causal notions of fairness are ubiquitous when trying to ensure equal treatment between social groups in algorithmic decision-making. In their FAT* 2020 paper, Lily Hu and CSI-affiliated researcher Issa Kohler-Hausmann argue that these traditional methods for ensuring fairness make a conceptual error by assuming that the membership in a demographic group is separable from the social phenomena that go along with it. Instead, they call for a reimagining of what mathematically formal definitions of fairness can be — and the normative assumptions that underlie them.
Paper: https://arxiv.org/abs/2006.01770
Blog post: https://www.computationsociety.org/2020/12/27/statistical-causal-fairness/